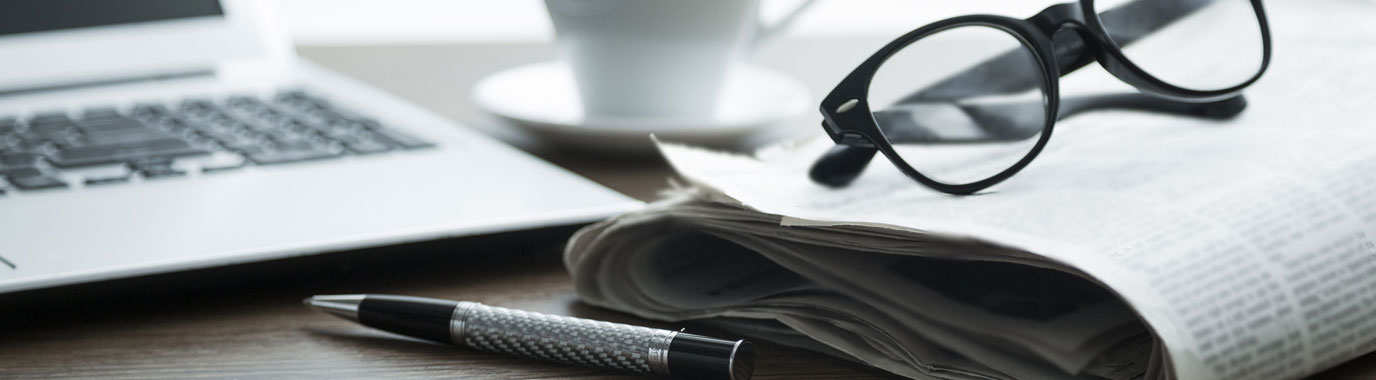
Explore our recent stories to see what drives us
How can artificial intelligence improve clinical trials?
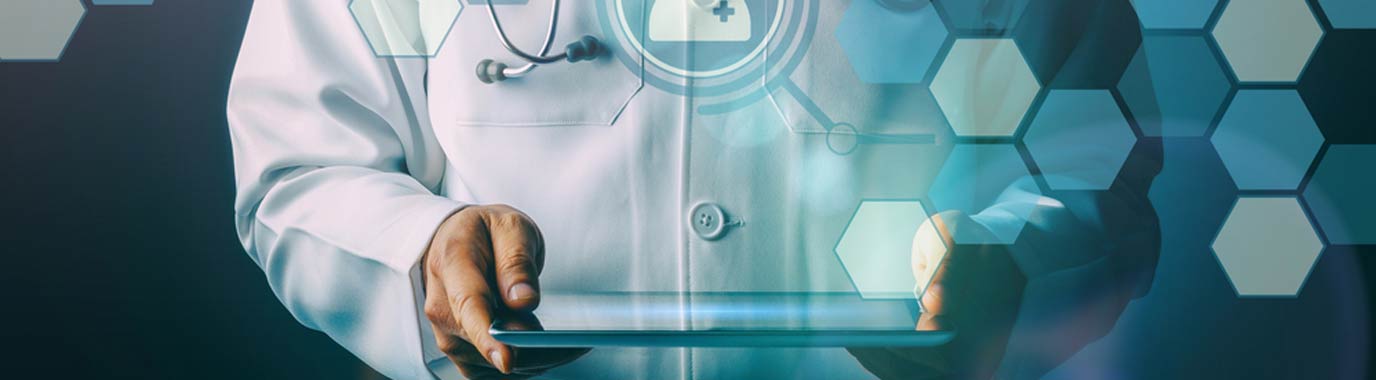
How are new digital technologies being used in clinical trials?
Yuri Martina: Machine learning and predictive analytics are able to make sense of large amounts of data much more quickly than a human ever could. This puts us in a stronger position to make accurate predictions about a range of issues that shape the success of a clinical trial – from deciding if, when and how a drug will work best, through to choosing which patients to include and predicting dropout rates, selecting a site, identifying and connecting the right patients to the right clinical trials, or defining the sample size and dosage.
What are the benefits?
Yuri Martina: It’s ultimately about increasing R&D productivity and becoming more patient-centric. Currently, only around 10 percent of drugs that are tested in human subjects receive FDA approval – and the research process can be extremely lengthy and cost millions of dollars. Many drugs fail because of inaccurate predictions which results in incorrect planning clinical developments and clinical trials. That’s why machine learning and predictive analytics are so exciting for our industry. They can help us improve trial design, accelerate the clinical development process and cut costs. And, most importantly, that means patients get access to better medicines sooner.
So machines will provide all the answers in the future?
Jorine Putter: Technology is not the answer to everything, human interaction is still a crucial part. And the outcomes of machine learning are only as good as the questions we ask and how well we’ve translated it into an analytical task: We need to make sure we’re looking at the correct unbiased data that has been categorised and organised accurately. Training this technology to achieve meaningful results will take some time, so we need to be patient. On top of this, companies need to invest in categorising and organising internal data, buying external data sets and setting up the required IT infrastructure. Success will rely on combining internal and external data and forming strong symbiotic partnerships, including cross-functional internal expertise and the right external partners.
To what extent are we already using these new technologies at Grünenthal?
Jorine Putter: We’re currently in the process of categorising and organising internal data. The next steps will include setting up a ‘data lake’ that also takes external data into account. Companies across the pharmaceutical industry are using machine learning and predictive analytics in different ways: There really are no limits to the potential range of insights it could generate in the future. We’re excited about how it will strengthen the way we plan and execute clinical trials – and determined to explore its full potential to improve patients’ lives.
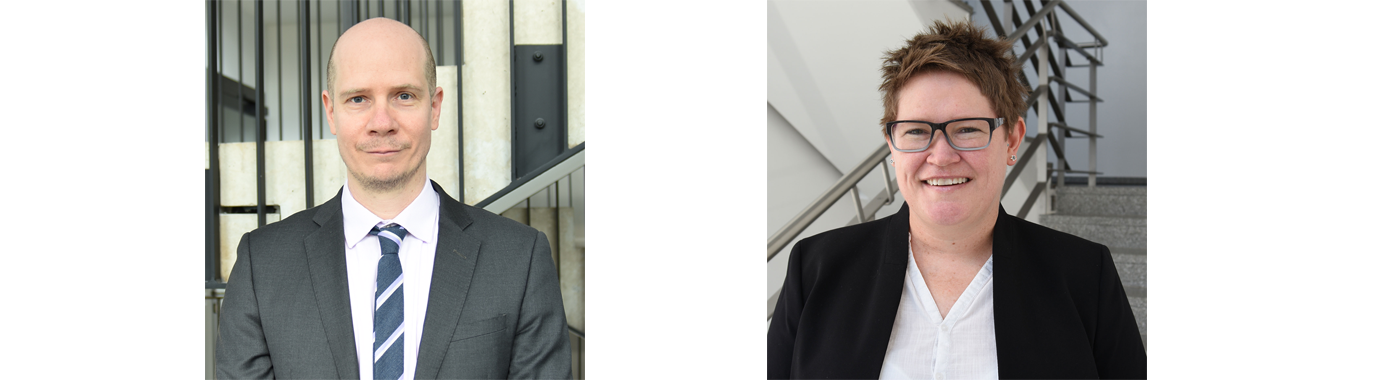
Predictive analytics can be defined as the procedure of condensing huge volumes of data into information that humans can understand and use. It examines data or content to answer the question “What is going to happen?” or more precisely, “What is likely to happen?”, and is characterised by techniques such as regression analysis, forecasting, multivariate statistics, pattern matching, predictive modeling, and forecasting.1
Machine learning (ML) is considered a modern-day extension of predictive analytics. It is a method of computational learning underlying most artificial intelligence (AI) applications. In ML, systems or algorithms improve themselves through data experience without relying on explicit programming. ML algorithms are wide-ranging tools capable of carrying out predictions while simultaneously learning from over trillions of observations. Efficient pattern recognition and self-learning are the backbones of ML models, which automatically evolve based on changing patterns in order to enable appropriate actions.2
1Gartner IT Glossary: https://www.gartner.com/it-glossary/predictive-analytics-2
2The Differences Between Machine Learning And Predictive Analytics. The Digitalist Magazine, 15 March 2018, https://www.digitalistmag.com/digital-economy/2018/03/15/differences-between-machine-learning-predictive-analytics-05977121