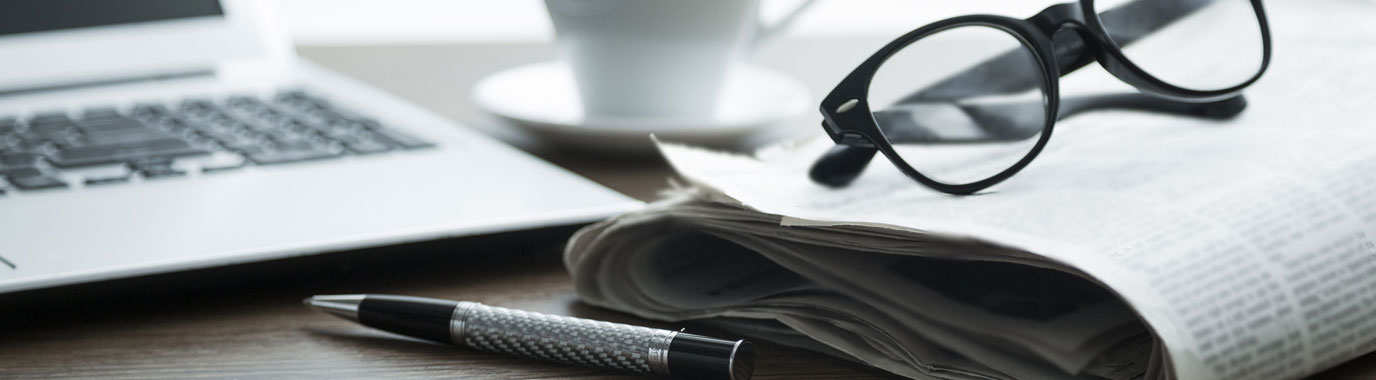
Explore our recent stories to see what drives us
Science Made Me develop algorithms that can design innovative molecules
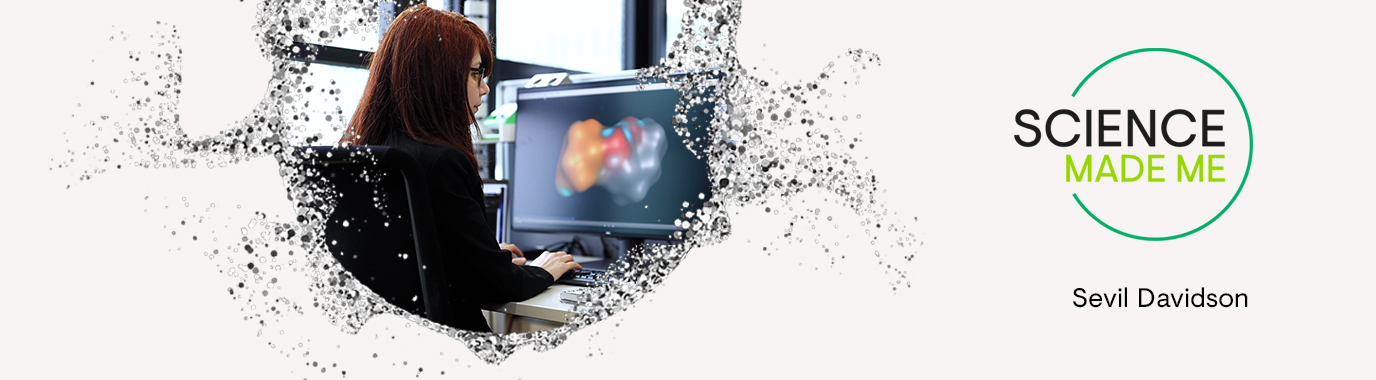
As one of Grünenthal’s Computational Biologists, Sevil Davidson contributes to achieving our vision of a world free of pain. Together with her colleagues, she leverages the opportunities digitalisation – especially artificial intelligence (AI) and machine learning (ML) – have brought to the life sciences. Sevil is a researcher through and through, already in high school it was clear that this would be her path when a project with a local university to investigate the bacterial biosynthesis of biodegradable plastic provided the decisive impulse for her professional career. It was in this very project, where she learned many of the key elements that still help her today – not being discouraged by setbacks and being unconventional in the way she pursues her goals. After succeeding to culture bacteria that can produce biodegradable plastic, Sevil went on to study molecular biology and then completed her doctorate in cellular biology. This was when Sevil found her passion for data and data analysis, which led her to do an additional Master's degree in machine learning.
Machine learning is a specific method of artificial intelligence, where large amounts of data are used to train algorithms to detect complex patterns and make classifications and predictions on this basis. This underlying mechanism of machine learning enables your mobile devices to recognise faces and learn to read your handwriting – which means making a correct assumption for each set of lines you put on the display. For software like “Alexa” and “Siri”, it provides the skills to understand voice commands and provide answers to your questions.
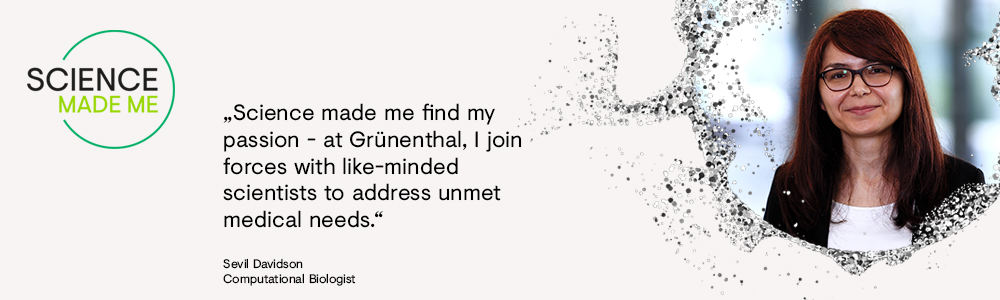
At Grünenthal, our computational scientists across R&D teams leverage opportunities from such technologies. However, Sevil, as part of this group, does not develop the algorithms to recognise faces or handwriting, she develops them to help drug development teams understand the relationship between the structural and physiochemical properties of molecules and thereby help design novel molecules with specific characteristics. Using information from existing chemical structures, Sevil trains a machine learning algorithm to predict certain properties of a molecule. Once trained, such an algorithm can be combined with further machine learning applications leveraged at Grünenthal to come up with thousands of new ideas on structures with enormous speed and thereby complement human expertise in the field. As Sevil and her colleagues run these in-silico approaches on a computer without the need to synthesize the molecules, they are efficient and can help accelerate experimental work. At the same time, they bring significant added value to the drug design process, providing the team the opportunity to iteratively analyse, design and adapt the molecule virtually until it has the desired properties.
For Sevil and her colleagues, it is the most satisfying feeling to finally have structures optimised by the machine learning drug design platform handed over to colleagues for synthesis in the lab for the first time. This is only surpassed when the data from the following pre-clinical experiments confirms what was calculated on the computer – that they may have been able to contribute to a new innovative medicine that could address the needs of patients around the globe.
Want to read more stories about our scienticsts? Check out the stories of Maria Stupar, Sarthak Sharma, and Dalena Brockwell.
Inspired by Sevil?
Check out the open positions in our Research & Development team on our careers website.
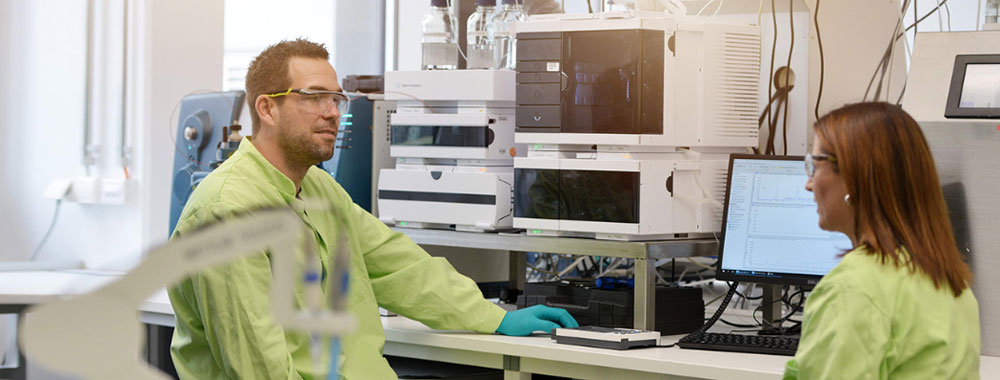